In the dynamic realm of technology, Natural Language Processing (NLP) has emerged as a groundbreaking field, revolutionizing the way machines understand and interact with human language.in this article, I tell you about the various examples of NLP, shedding light on its applications, challenges, and the transformative impact it has on diverse industries.
Understanding the Basics of NLP
What is Natural Language Processing?
Natural Language Processing is a subfield of artificial intelligence (AI) that focuses on the interaction between computers and human language. It enables machines to comprehend, interpret, and generate human-like text, fostering a seamless interface between humans and technology.
Core Components of NLP
- Tokenization: Breaking down text into smaller units, such as words or phrases, to facilitate analysis.
- Part-of-Speech Tagging: Identifying the grammatical parts of words, like nouns, verbs, or adjectives.
- Named Entity Recognition (NER): Extracting specific information such as names, locations, and dates from text.
- Sentiment Analysis: Evaluating the emotional tone expressed in a piece of text, be it positive, negative, or neutral.
Examples of Natural Language Processing (NLP)
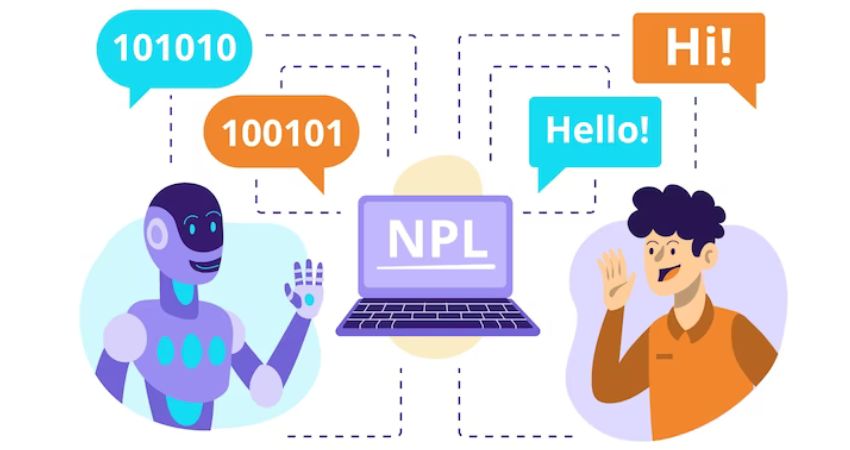
Natural Language Processing (NLP) is a field concerned with making computers understand, interpret, generate, and manipulate human languages. Here are ten examples showcasing various NLP applications:
- Speech Recognition: Systems like Amazon Alexa, Google Assistant, Apple Siri, and Microsoft Cortana convert spoken words into written text and execute commands accordingly.
- Text Summarization: Creating concise summaries of long documents or multiple articles on the same topic, e.g., news aggregators or research paper synopses.
- Sentiment Analysis: Analyzing opinions expressed in textual form to determine overall sentiment, used extensively in social media monitoring, customer feedback management, and brand reputation tracking.
- Spell Checking & Grammar Correction: Tools integrated into word processors, email clients, and messaging platforms that automatically detect and correct spelling mistakes and grammatical errors.
- Language Translation: Automated translations of web pages, chatbots, multilingual websites, and real-time translation services provided by tools like Google Translate and Skype Translator.
- Chatbots & Virtual Assistants: Implementing conversational interfaces for businesses and organizations to interact with customers, provide support, answer queries, and automate routine tasks.
- Information Extraction: Identifying structured information from unstructured sources, such as mining contact details from emails, gathering company information from annual reports, or scraping product reviews from online stores.
- Topic Modeling: Discovering hidden themes present in collections of documents, useful for categorizing content, clustering search results, or identifying emerging trends.
- Part-Of-Speech Tagging: Labeling individual words in sentences according to their syntactic roles, enabling a better understanding of sentence structure, semantic relationships, and meaning extraction.
- Question Answering Systems: Providing precise answers to questions posed in natural language format, seen in virtual assistants, FAQs, educational resources, and trivia games.
These examples demonstrate the wide-ranging applicability of NLP technologies in everyday life and industry sectors, enhancing communication efficiency, user experience, and automated workflows.
Challenges in NLP
Natural Language Processing (NLP) faces several challenges that arise from the complexity and variability of human language. Some of the key challenges in NLP include:
Ambiguity in Language:
- Issue: Human language is inherently ambiguous, and words or phrases can have multiple meanings depending on context.
- Impact: NLP systems may struggle to accurately interpret and understand the intended meaning of a sentence or phrase without sufficient contextual information.
Cultural and Linguistic Variations:
- Issue: Different languages and dialects, as well as variations in cultural expressions, present challenges for NLP models that may not generalize well across diverse linguistic landscapes.
- Impact: NLP systems may exhibit reduced accuracy when processing languages or cultural contexts they were not specifically trained on.
Handling Sarcasm and Irony:
- Issue: Sarcasm and irony rely heavily on context and tone, making them challenging for NLP models to accurately identify and comprehend.
- Impact: Misinterpretation of sarcastic or ironic statements can lead to inaccurate results in sentiment analysis or natural language understanding.
Bias in NLP Models:
- Issue: NLP models can inadvertently learn and perpetuate biases present in the training data, leading to unfair or discriminatory outcomes.
- Impact: Biases in NLP can result in biased recommendations, reinforcing stereotypes, and contributing to inequitable representations in automated systems.
Limited Context Understanding:
- Issue: NLP models often struggle with understanding the broader context of a conversation or document, especially when dealing with long or complex pieces of text.
- Impact: Lack of contextual awareness may result in misinterpretations and hinder the ability to generate accurate and relevant responses.
Data Quality and Quantity:
- Issue: NLP models heavily rely on large and diverse datasets for training, and the quality and representativeness of the data can significantly impact model performance.
- Impact: Inadequate or biased training data may lead to suboptimal performance and may not generalize well to real-world scenarios.
Dynamic Nature of Language:
- Issue: Language is constantly evolving with new words, phrases, and expressions emerging over time.
- Impact: NLP models may struggle to keep up with these changes, affecting their ability to understand and generate text that aligns with current linguistic trends.
Lack of Explainability:
- Issue: Many NLP models, especially deep learning models, can be complex and challenging to interpret, leading to a lack of transparency in their decision-making processes.
- Impact: Understanding why a model made a specific prediction or decision is crucial for gaining user trust and ensuring accountability, especially in sensitive applications like healthcare or finance.
Addressing these challenges requires ongoing research and development efforts to enhance the robustness, accuracy, and ethical considerations of NLP systems.
How does NLP benefit businesses?
NLP benefits businesses by enabling sentiment analysis, improving customer interactions through chatbots, facilitating multilingual communication, and aiding in data summarization for informed decision-making.
Can NLP understand slang and colloquial language?
NLP models are continually improving in understanding slang and colloquial language, but challenges remain due to the ever-evolving nature of informal expressions.
What are the key challenges in implementing NLP?
Challenges include dealing with language ambiguity, addressing cultural and linguistic variations, handling sarcasm and irony, and mitigating biases present in NLP models.
How can businesses mitigate biases in NLP models?
Businesses can mitigate biases by using diverse and representative training data, regularly auditing models for fairness, and implementing ethical guidelines in NLP development and deployment.